Confidence data plays a crucial role in various fields, from statistics to psychology. It quantifies the level of certainty associated with measurements, predictions, or decisions, providing a clearer picture of reliability. By understanding how to interpret confidence data, I can make more informed choices in daily life and professional scenarios.
In the realm of data analysis, confidence levels help assess how much trust I can place in calculated results, especially when making predictions or drawing conclusions. This understanding empowers me to weigh options more effectively and recognize the limits of the information presented.
Exploring the intricacies of confidence data not only enhances my analytical skills but also fosters better decision-making. I find that grasping the fundamentals of this concept opens up a wealth of knowledge applicable in various personal and professional situations.
Understanding Confidence Data
Confidence data provides insights into the certainty associated with various data points and predictions. This information is crucial for decision-making processes across multiple fields.
Definition and Importance
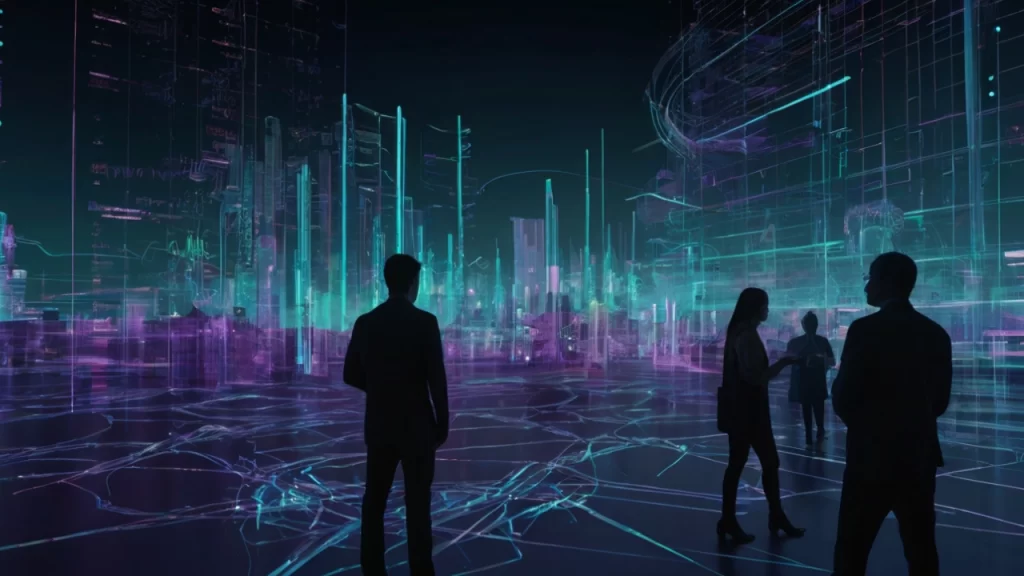
Confidence data refers to statistical measures that indicate the reliability and certainty of a given estimate or prediction. It is commonly expressed through confidence intervals or confidence scores.
A confidence interval, for example, provides a range within which we expect the true value to fall with a certain probability. This helps quantify uncertainty in estimations, making it a vital tool in fields such as finance, science, and market research.
Importance lies in its ability to guide informed decisions. The clearer the confidence data, the more accurately I can assess risk, make forecasts, and draw conclusions. In business, having reliable confidence data can lead to better strategies and improved outcomes.
Sources of Confidence Data
Confidence data can be derived from various sources, each offering distinct advantages. One primary source is statistical analysis, where I analyze historical data through methods like regression analysis or hypothesis testing.
Surveys and experiments are another source. I can conduct surveys to gather quantitative data reflecting public opinion or user behavior. The design and sample size of these surveys critically impact the confidence data generated.
Additionally, data from governmental and institutional reports provide reliable benchmarks. These reports often contain aggregated data that I can use for comparison and analysis. Utilizing diverse sources strengthens the validity of the confidence data I rely on for decision-making.
Applications of Confidence Data
Confidence data plays a crucial role in various fields by providing insights that enhance decision-making processes, user engagement, and risk management. Understanding its applications helps identify the benefits it can bring to businesses and individuals alike.
Business Decision Making
In business, confidence data can significantly influence strategy formulation. I utilize this data to gauge market trends and consumer preferences. By analyzing patterns and statistical likelihoods, I can make informed choices that align with organizational goals.
For example, A/B testing results combined with confidence intervals allow me to determine which product variations resonate best with consumers. This approach helps in resource allocation, ensuring that investments are directed toward initiatives with the highest potential for success.
Improving User Experience
Confidence data serves as a valuable tool for enhancing user experience across platforms. By collecting and analyzing user interactions, I can identify which features are most effective and which require improvement.
Using confidence metrics from user surveys, I assess satisfaction levels and recommend targeted enhancements. For instance, if a specific navigational feature receives low confidence scores, I can prioritize its redesign. This targeted approach fosters user retention and satisfaction.
Risk Assessment
In risk management, confidence data helps in evaluating potential threats and opportunities. I apply confidence intervals to forecast various outcomes, enabling more accurate risk profiles.
For instance, I examine historical data trends to assess the likelihood of product failure or market fluctuations. By quantifying this uncertainty, I can develop strategies that mitigate potential risks while capitalizing on favorable conditions. This analytical framework is critical for creating resilient business models.
Measuring and Analyzing Confidence Data
In assessing confidence data, I focus on two primary approaches: quantitative methods and qualitative techniques. Each method provides unique insights, helping to understand behaviors and perceptions regarding confidence.
Quantitative Methods
Quantitative analysis involves numerical data, providing measurable insights into confidence levels. I typically employ surveys with Likert scale questions to gather data. This scales participants’ confidence from, for example, 1 (not confident) to 5 (very confident).
Data can be analyzed using statistical methods. Common techniques include:
- Descriptive statistics: Mean, median, mode, and standard deviation summarize confidence levels.
- Inferential statistics: T-tests or ANOVA can analyze differences among groups.
Visualization tools, like scatter plots or bar charts, help illustrate findings and trends effectively.
Qualitative Techniques
Qualitative methods provide depth to understanding confidence. I often use interviews or focus groups to gather detailed perspectives. These discussions allow me to explore how individuals perceive and express confidence.
Key techniques include:
- Thematic analysis: Identifying patterns or themes within responses.
- Content analysis: Examining the frequency of specific words or phrases related to confidence.
These approaches help capture nuanced views. I can then compare qualitative insights with quantitative findings to achieve a comprehensive understanding of confidence data.
Challenges and Considerations
When addressing confidence data, several challenges and considerations arise that require careful thought. Ensuring reliability and recognizing ethical implications are crucial in effectively utilizing this data.
Data Reliability and Validity
I encounter significant issues related to data reliability and validity when working with confidence data. This aspect is critical because unreliable data can lead to incorrect conclusions.
Factors affecting reliability include sample size, data collection methods, and potential biases. For instance:
- Sample Size: Smaller samples may exaggerate variability, impacting confidence estimates.
- Data Collection Methods: Surveys or experiments must be robust to avoid skewed results.
- Bias Factors: Selection bias can diminish the credibility of outcomes.
To enhance validity, I must ensure that confidence measures accurately reflect what they intend to assess. This involves rigorous testing of assumptions and techniques during the data gathering process.
Ethical Implications
Ethical considerations are paramount in the collection and application of confidence data. I have to be mindful of the potential consequences of misusing this data, which can lead to harmful outcomes.
Key ethical issues include:
- Informed Consent: Ensuring participants understand how their data will be used.
- Data Privacy: Protecting the confidentiality of individuals while analyzing aggregate data.
- Misrepresentation: Avoiding the manipulation of confidence levels to mislead stakeholders.
I should develop transparent methodologies and uphold high ethical standards in research. This approach fosters trust and integrity among participants and audiences alike.